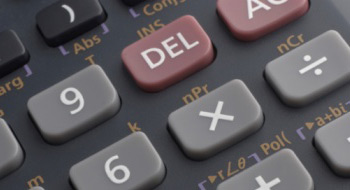
Are you interested in forecasting your pension plan’s funded position? If so, read on.
In fact, you can even determine your plan’s expected average contributions and funded position just by having an idea of what average inflation or investment returns to expect over the next few years.
Still intrigued?
Stochastic modelling—estimating potential outcomes by allowing for random variation in one or more inputs over a period of time—allows pension plans to do just that.
However, in the process of forecasting your plan’s funded position, you’ll have to consider the following questions:
• How high or low could inflation and investment returns end up being over the next few years?
• How strongly or poorly funded could your plan become at the end of, and during, those years?
• How can you determine a credible range of possible future funded positions?
Stochastic modelling can help you tackle these issues.
By projecting many possible future paths of inflation, investment returns and interest rates (some higher, others lower), plans can model changes to both assets and liabilities. This results in many possible future outcomes of a plan’s financial position. Therefore, you can calculate the likelihood of attaining certain financial objectives.
Getting there is important
Focus should not be placed exclusively on the outcome of the plan’s financial position at the end of the projection period. Just as important is examining the path the plan’s financial position follows during the course of an individual trial.
In Figure 1, considering all scenarios together, there’s a 75% chance that after 30 years, the plan would end above 100% funded. However, just as important is determining how many of those trials went through years of low returns resulting in a poor financial position.
For example, the chart’s dotted black line highlights one of the many trials within a typical stochastic analysis. Trial 5 deteriorates to a low of 62% funded after eight years, before recovering to finish above 100% after 30 years.
Would trustees of this plan realistically maintain their composure when the plan is in a dire underfunded position at year eight by sticking to the plan’s original investment policy? Would trustees move to a more conservative investment policy having grown a distaste for the market’s risk? Would there be benefit cuts to shore up the plan’s position?
If the trustees change the investment policy, the results of the stochastic modelling risk study are no longer valid for subsequent years. Therefore, the study’s results will be drastically affected by considering the path of each trial in addition to its ending financial position. Stochastic modelling works best when both the journey and the destination matter.
One trial
Trustees may mistake the precision of stochastic modelling results as having equivalent accuracy to real-life future outcomes. If the model shows a 25% chance of a plan becoming underfunded after 10 years, there’s ultimately no way of proving this probability.
Contrast this to flipping a coin. You can predict that tails will turn up 50% of the time. And you can test this hypothesis by flipping a coin many times and counting the number of tails. But, by saying a pension plan has a 25% chance of becoming underfunded after 10 years, you can’t play out thousands of trials in real life; reality provides only one actual trial.
If the plan, in fact, does become underfunded, you can’t say this one actuarial trial of reality fell within those 25% of underfunded trials, nor can you say the model was accurate. Even if a robust model can calculate probabilities with great precision, it doesn’t imply that those calculated probabilities are accurate in real life.
Stochastic modelling can provide insight into a range of possible outcomes rather than just an expected or mean outcome. However, you have to be careful how you interpret these results. Do the trials really represent real-world situations?
Additionally, trustees should consider downside trials even if their chance is minimal, because one of these downside trials could end up being the actual outcome of the pension plan.
This highlights the importance of stress testing. Extreme downside trials in stochastic modelling are often disregarded as outliers. In contrast, stress testing forces consideration of what effect an extreme and immediate shock to economic variables, such as equity movements or interest rates, can have on a plan’s funded position.
Setting assumptions
A stochastic model’s assumptions ultimately drive its results.
The recent analysis done for New Brunswick’s shared-risk pension model illustrates this well. The original task force that advised transitioning to this shared-risk pension model in 2014 concluded that the chance of a cut to base benefits over the next 20 years was a negligible 2.5%. However, PBI’s review determined the chance was substantially higher, at 27.5%.
Different results
Although the task force and PBI used essentially the same stochastic model, PBI used a reasonable but lower investment return assumption than that of the task force. This seemingly small difference drove the drastically different result.
If changing this input from one reasonable assumption to another affected the results to such a degree, can you imagine what the results would be if the modelling assumptions weren’t reasonable at all? Even the most sophisticated stochastic model will produce misleading results if you’re not careful when you set the inputs.
Stochastic modelling can be a helpful tool in a pension plan’s decision-making process because it provides an understanding of the range of possible outcomes and the sensitivity of those outcomes, especially when assumptions are changed. However, relying too heavily on stochastic modelling can create a false sense of accuracy and a tendency for trustees to be less prepared to address downside risk and short-term volatility.
Just like any analytical tool, stochastic modelling has limitations. Its analysis should be only one of trustees’ considerations when making a decision.
Julia Friesen is an investment analyst with PBI Actuarial Consultants Ltd. info@pbiactuarial.ca