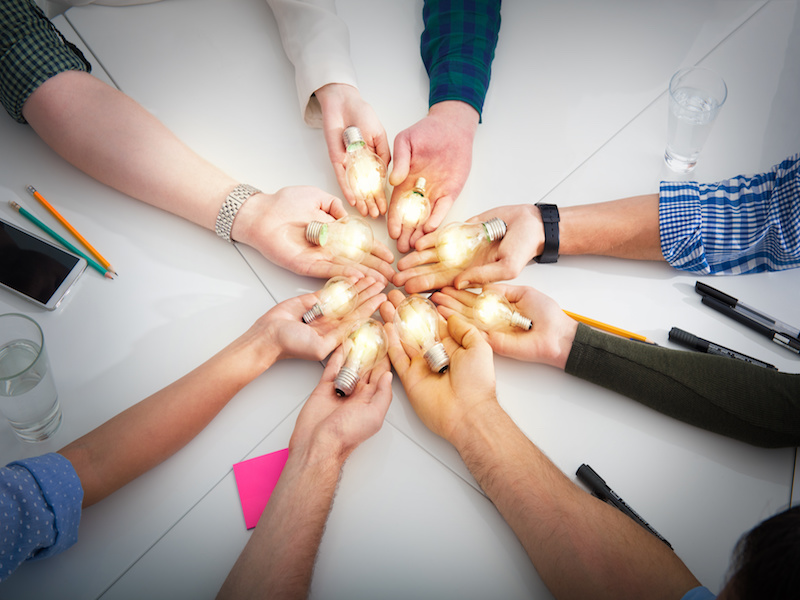
There’s a crisis in financial research because of statistical shortfalls, says Marcos López de Prado, a professor of practice at Cornell University’s school of engineering and chief investment officer of True Positive Technologies.
“When a journal receives a paper, they don’t know how many experiments the researcher has conducted and, as a result, they cannot account for the possibility that the discovery is a statistical fluke and it’s just by luck,” he says.
An alternative to traditional research is using crowdsourcing techniques, he says, which is the topic of a recent paper he co-authored. Set to be published in the Journal of Financial Data Science, the paper outlined barriers to traditional financial research and how crowdsourcing can help overcome each of these barriers, either through investment tournaments or developing back-testing platforms.
One barrier to investment research is that data isn’t available because it’s proprietary or expensive. “This is a problem because it means that, on one hand, the large majority of the research community is not able to look into the data and explore new ideas. And so that’s one problem. And the second problem is that the people who have access to the data may only be looking at ideas that they have some preconceptions [about].”
Another barrier is that modelling data requires domain-specific knowledge, which only exists among a small number of researchers, says López de Prado. “So as a result, again, what happens is that most investment opportunities are not being discovered because there are very few people who, No. 1, have access to the data and, No. 2, even if they have access to the data, even fewer people know how to model this data and what the data means.”
In a platform approach, an investment manager can post data on a website or platform and the entire scientific community can work on the problem. This can enable undirected crowdsourcing where many researchers have the data to back-test various investment strategies.
The platform approach solves the problem of open data, but pitfalls still exist, says López de Prado. “The negative is that . . . platforms require that every researcher must be an expert. Because the data’s provided as is and that means that the data scientist who, perhaps doesn’t have a background in finance, now must deal with data sets that are very specific, very characteristic of finance.”
The platform approach also makes it difficult to control for back-test overfitting so there’s a high probability of false positives, he says. And it may not prevent selection bias.
In a tournament approach, on the other hand, the data is transformed into a well-defined investment problem, so that researchers have access to the data but can only use it in the context of that specific problem. This enables directed crowdsourcing, the paper said.
The approach also overcomes the data problem and the knowledge barrier, says López de Prado. And, it allows any data scientists to participate.
“Instead of it being solved by a small club of researchers, that [data] can be posted to the entire population of scientists around the world who can work on these models, who are already very familiar with these techniques, people who have been doing research on all sorts of things . . . They already know these models, they receive these data sets and they’re already presented in a way that they do not need to have financial knowledge. So that’s where we are able to essentially democratize investment research.”
And tournaments can also solve the problem of back-test overfitting because organizers provide the data in an obfuscated way, so they can control what the researchers have access to. “Because the researcher does not have access to the test set, selection bias is prevented.”
Investment research tournaments are already taking place through online platforms, where an investment firm can pose a problem and offer a reward.
Investment firms are also hiring data scientists, notes López de Prado, because there’s a tremendous amount of data that wasn’t available three years ago. “And dealing with this data requires knowledge with algorithms and the ability to run super computers and be knowledgeable in machine learning.”
But there’s still a role for people with a finance background, which is transforming data sets into a financial problem, he says. “And this data transformation requires tremendous experience and understanding of how the markets work.”
Tournaments are a way of dividing tasks between finance and data science, says López de Prado.
This is significant because it can help firms reduce fees, so instead of employing 200 people, they can pose problems to the entire scientific community.
“How do you reduce fees? Well, through automation and crowdsourcing. So that’s why crowdsourcing is becoming very quickly accepted, because there is no choice anymore. Investment firms need to automate and research must be crowdsourced.”
It’s also effective because markets are inefficient, says López de Prado, and in order to capture inefficiencies, investment firms must modernize.
“This is a case of microscopic goals. As you know, today, in any given year, worldwide, the amount of gold that is being extracted is a large multiple of the amount of gold that would be extracted in the 16th century. Why? Because of chemical processes and industrialized production. The same happens with the extraction of alpha today in 2019. The alpha is there —today there is more alpha than there was in 2010. It’s just that it requires sophisticated techniques to be extracted.”