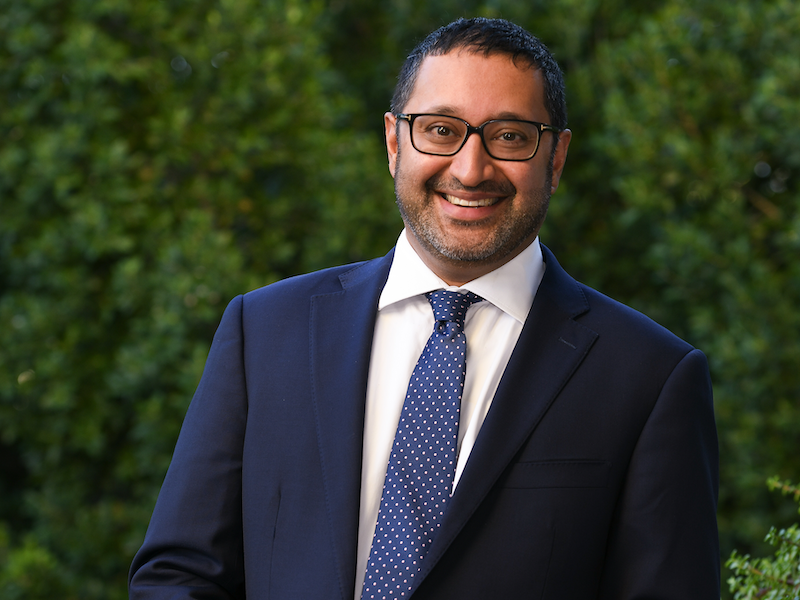
Since interpretations of environmental, social and governance investing can vary, institutional investors can leverage artificial intelligence tools to strip away the noise and reveal data sets required to eliminate greenwashing and generate sustainable alpha, said Andy Moniz, senior vice-president and director of responsible investing at Acadian Asset Management, during the Canadian Investment Review’s 2022 Investment Innovation Conference.
For ESG to be a source of alpha, investors must overlook or under-react to certain standard elements of ESG information, such as typical quarterly earnings reports and long-term sustainability information, he said, noting that, at some point, elements of ESG information must manifest into any prospective company’s earnings.
“It’s extremely difficult to collect, process and decipher ESG information in an objective and scalable way and that’s precisely why we need machine learning techniques.”
Read: How AIMCo is building a scalable artificial intelligence program
Acadian’s own ESG ratings are based on unstructured data and machine learning techniques. The organization pulls this qualitative information from companies’ sustainability reports, regulatory filings, patents and earning score transcripts, automatically extracting patterns from data.
Using natural language processing techniques, Acadian is able to automatically read various documents and extract information to identify other affiliate businesses mentioned in news articles, said Moniz, noting these algorithms can be used to automatically extract information for 40,000 securities globally to identify complex customer/supply relationships for every company.
Acadian’s algorithm can also analyze market price information, recognizing cases in which regulators may have imposed a windfall tax or carbon tax on energy companies or those that received subsidies for alternative energy. “We use this algorithm . . . to automatically identify escalating ESG risks. . . . We’ll add stocks into a no-buy list so that we don’t buy further into [companies with] these reputational concerns. We [also] use this algorithm for engagement work because it allows us to automatically identify . . . trending ESG issues . . . we should be thinking about.”
Typically, the criticism is that it’s very difficult to engage with a discretionary manager that holds a concentrated portfolio in order to meet them and ask questions, said Moniz. “Because we hold large portfolios, . . . it simply isn’t feasible to meet every company and company management. We need other techniques to screen our portfolios before we pick up the phone and actually engage with companies.”
Read: Tips for avoiding greenwashed investments and service providers
Deep learning is nother technique Acadian uses to determine the ESG ratings of prospective companies. Its natural language processing algorithms are trained on 3.3 billion combinations of words and how they’re being used in company sustainability reports and all other sources. “One of the outputs is this word cloud [that] you can think of . . . as . . . an ESG thesaurus,” he said. “This algorithm automatically places words in this cloud in such a way that words that are next to each other are deemed to be semantically similar, highlighting words such as Paris, science-based, ambitions, goals, targets [and] climate neutrality. The algorithm, by processing billions of combinations of words, has learned that, typically, companies use these words when they’re talking about decarbonization plans [and is] looking at a vast amount of data to understand these co-occurrences.”
The model is even able to detect greenwashing in companies by deciphering to what extent a company is talking about ESG issues, such as decarbonization, and measuring its actual behaviour, noted Moniz. He showed a comparison of two companies, one for which the algorithm read through earnings call transcripts, picking up linguistic cues in sentences that note ‘they may possibly consider decarbonization in the future” or “it may aim to look at Scope 3” without stating actual commitments or targets. The other company was very transparent with granular disclosure language that included catch words, such as numeric information, baselines, targets and percentage reductions.
“Our algorithm can automatically identify this across languages for 40,000 securities globally . . . [and] can identify . . . the winners and the losers and then infer what it means in terms of a return forecast going forward for every company in this network.”
Read more coverage of the 2022 Investment Innovation Conference.