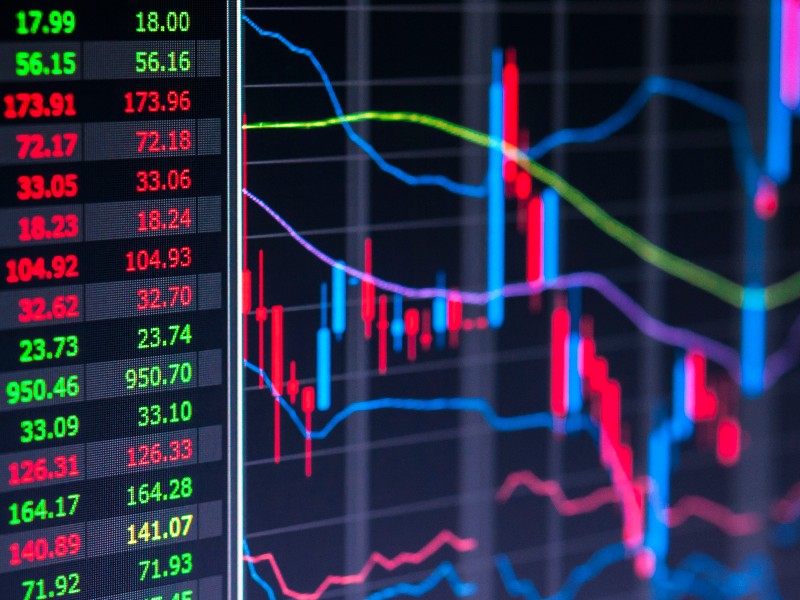
Chief financial officers may be over-confident when it comes to investment forecasts, even when they’ve been proven wrong in the past, according to a new paper by researchers at Duke University and Ohio State University.
“Senior financial executives’ beliefs of the mean of the distribution of S&P 500 returns are accurate, but they appear to systematically underestimate the variance of this distribution,” the paper said. “We refer to this as miscalibration, a type of overconfidence.”
The researchers looked at 14,800 CFO forecasts of one-year S&P 500 returns over a 12-year period and also collected the respondents’ confidence levels to gauge their expected return volatility using results from Duke University’s quarterly CFO survey results from the second quarter of 2001 to the third quarter of 2017. “We find that the average CFO forecast of the return is approximately five per cent and the average realized annual return is also five per cent over the same period,” the paper said. “On the other hand, the standard deviation of realized annual returns is 17.1 per cent, while the average of CFOs’ beliefs of the standard deviation is only 5.5 per cent, less than one-third of the historical experience.”
In the survey, CFOs provided 80 per cent confidence intervals for their forecasts, gauging their beliefs on expected return volatility. When looking at the full sample, the research found CFOs hit their 80 per cent confidence level only 31.5 per cent of the time, implying extreme overconfidence in their forecasting abilities.
In particular, the paper examined individuals who provide multiple forecasts to see how they learn from their mistakes. “Many executives provide multiple predictions over time, i.e., after having observed the realization of their prior forecast,” it said. “This setting allows us to measure how these executives update their beliefs. Our main finding is that when the realized return falls outside an executive’s confidence interval, their subsequent confidence interval widens. While the widening of intervals is economically and significant, the size of the widening is insufficient to obtain proper calibration and, as a result, miscalibration persists.”
And although confidence levels do widen with repeated misses, the changes are small and decrease with both experience and initial miscalibration, the paper found. “We observe that CFOs learn and argue that the slow updating is consistent with very strong prior beliefs about the variance process. CFOs who miss the interval update on average by approximately 7.5 [percentage points], but would need to update by an additional 23.1 [percentage points] to attain proper calibration. With enough time, our framework implies the CFOs should obtain proper calibration. However, even the forecasters with the longest track records do not converge.”
The paper was written by Michael Boutros, John R. Graham, Campbell Harvey and John Payne from Duke University and Itzhak Ben-David from the Ohio State University.