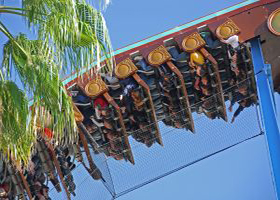
One of us recently gave a presentation entitled “The Right Answers to the Wrong Questions”, which was subsequently the subject of a Canadian Investment Review blog post by Tris Lett. The presentation, and the book we are writing with the same title, traces the emergence of the branch of probability and statistics called Extreme Value Theory in parallel with models of prices in mathematical finance over the course of the 20th Century. This history reveals a number of missed opportunities which, if taken, would have changed the course of finance and in particular the course of financial crises.
One of the most crucial of these was (and still is) the failure of the finance industry to make use of Expected Shortfall and Extreme Value Theory to manage the risk of large losses–something the insurance industry has been doing for several decades.
Here’s how it would have affected a manager with $10 million invested in Citigroup shares at the end of January 2007. In the previous 250 days, the worst one day loss on the Citigroup position was 2.47% or $247,000. How much worse could a 1-day loss be? While it is impossible to put a bound on this (aside from the knowledge that a stock price can go to zero), there is a useful answer, on average. Expected Shortfall, conditional on a loss of more than 2.47%, is the average of all returns below -2.47%.
By our calculations such an event could have been expected once in 136 days and the average of such losses would be 3.73%. In other words, our analysis said that a worse result was quite likely and that on average it would be about half again as great as the previous low, exposing the manager to a loss of $373,000. Armed with this information, he could have managed the risk by re-sizing the position if necessary.
Simple enough. But surely you can only calculate Expected Shortfall if you have a model for the Citigroup returns? Fortunately, you only need a model of the tail of the Citigroup returns, a much more tractable problem.
This is one of the lessons of Extreme Value Theory (EVT): the extreme events depend only on the tails of the distribution and must be studied in their own right. This is a very good thing indeed as the search for an adequate model of financial returns is a problem which might never be solved. By contrast, good models of tails of returns distributions have been available for more than thirty years.
The EVT story began in 1928 with a landmark paper by statisticians Ronald Fisher and L.H.C. Tippett. They showed that the largest or smallest values in a sample of independent draws from a given distribution could only behave in three ways, regardless of what distribution the samples were taken from.
We can give a rough summary of their results by saying that the nature of these extreme values depends only on a number now called the ‘shape parameter’. (While it was by no means obvious at the time, it eventually became clear that only one of the three types of distributions discovered by Fisher and Tippett was appropriate in finance and the shape parameter in this case is essentially a count of the number of finite moments. The limiting case of an infinite shape parameter is the case which describes the extremes of, for example, a Normal distribution. Typical financial data, on the other hand, corresponds to shape parameters in the range from 2 to 5–vastly different from Normal.)
The smaller the shape parameter, the ‘fatter’ the tail and the greater the propensity for extreme events. The contrast is quite apparent in the Citigroup example. According to a Normal distribution model the frequency of a loss of at least 2.47% was only 1 day in 717 and the average such loss was 2.71%.
On 27 February 2007 Citigroup lost 3.93% so the manager’s position was down $393,000. This was only $20,000 worse than the EVT estimate but it was $122,000 worse than the Normal model’s prediction. The contrast between the EVT and Normal estimates is entirely typical. The EVT model predicts much more frequent and much larger losses than the Normal model–exactly what is observed in financial markets.
While Fisher and Tippett’s discovery was made well in advance of the 1929 Crash, it couldn’t have contributed much to risk management in that crisis, even if investors had been making statistical studies of price movements. There was a catch. Fisher and Tippett had no general method for determining the shape parameter for a given distribution. That would take another 15 years to emerge in a paper by Igor Gnedenko.
Even after Gnedenko’s discoveries, determining the shape parameter from data, rather than from a formula for the distribution, was a problem that would take a further thirty years to solve. In 1975 James Picklands III published a paper that contained statistical tests to determine the shape parameter. He also provided an effective tail model which approximated the Extreme Value Distribution with that shape. With Picklands’ results, the modern era of EVT began.
We have recently developed highly efficient new statistical tools with which to implement EVT tail models. In the next installment of this article we travel back in time to January 1929 to see how, armed with our new technology, a US equity investor would have fared in the run-up to the 1929 Crash.
Shadwick and Cascon’s series on Extreme Value Theory will continue next week.
Ana Cascon and William F. Shadwick are with Omega Analysis Limited in London, England. Their Book, The Right Answers To The Wrong Questions, is represented by Sophie Hicks at the Ed Victor Agency, London.