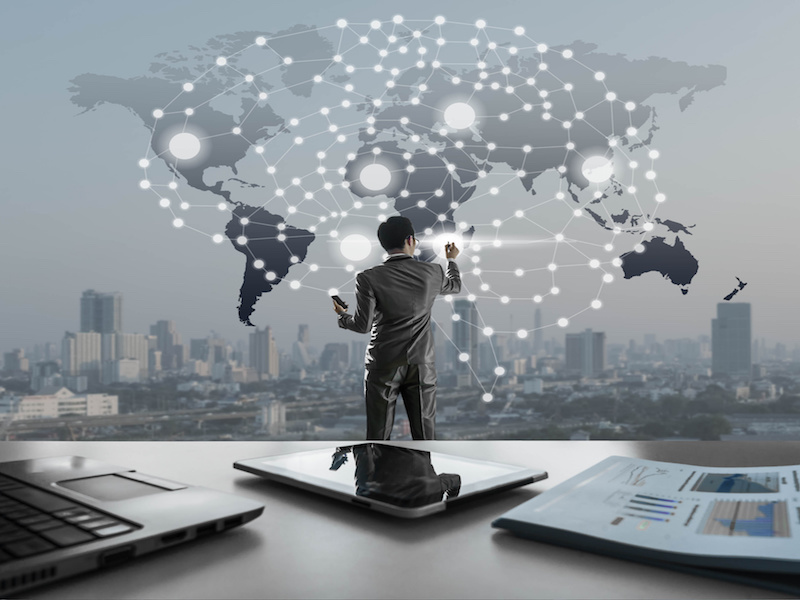
Active investors should combine their own analytical work with the use of machine learning to get a leg up on their passive peers, according to a new paper.
Fiona Frick, Unigestion’s chief executive officer, argued that, in an environment where institutional investors are using the same strategies and no one is left with an edge, active managers should use “collaborative intelligence” to generate alpha.
“We believe machine learning makes a lot of sense at the beginning of the investment process [as a] filter,” says Frick. “And then you can concentrate, as investment managers, on the next step, once the filtering has been done.”
Read: Institutional investors turning to AI, data science to improve processes, yield alpha
In the paper, Frick noted machine-learning models can help investors process vast amounts of data more quickly and regularly, while reducing subjectivity and individual bias.
However, these models can also have flaws. For example, they’re “inherently backward-looking,” which is why it’s important to avoid over-relying on machines and instead pair their analyses with investment managers’ ability to interpret new developments in the market.
“Machine learning can be very good to estimate in the current environment — [whether we’re] more in a recession or inflation mode — but things happen in the market, like geopolitical tensions, which are not well-reflected in past history,” says Frick. “That’s where you need an investment manager. . . . [They’re] very good at imagining a future,”
Investment managers can also provide “economic meaning” to the correlations drawn by machine learning algorithms, she argues. While models can discover relevant and powerful new connections in the data, they can also draw lines between unrelated factors or unveil biases in the data itself.
“Typically, the machine will tell you, for one reason or another, there are some stocks that move together and therefore there is a factor that moves them together. Perhaps that’s true and you haven’t seen it — because we are in a market that, in certain sectors, correlate together because of a macroeconomical scenario — and perhaps it’s not. It’s just a curious correlation.”
Read: CPPIB, PSP part of group investing in new AI fund
Rather than using machine learning for “short-term alpha signals” in liquid assets such as public equities, it’s possible to use models to find patterns that help refine its risk measurements, screening out primary funds in private equity that don’t fit its characteristics and adapting its portfolios to new signals. Models can also use machine learning to analyze macroeconomic sentiment indicators from traditional news and social media.
For risk assessment in macroeconomic scenarios, different risk measures make sense at different times, says Frick, “and we can use machine learning to help define which to give more weight at one point in time.”
The technology could also be a useful tool for managing and interpreting environmental, social and governance data. “ESG relies on a lot of data that’s not in a classical database. . . . A lot of [that] data comes from news [stories], which are not necessarily things we’ve used in the past,” she notes. “It’s very useful to use all these new alternative sources of knowledge about a company, a sector, a country, etc., but you need some power behind that . . . and that’s where machine learning helps.”
Read: AIMCo enters partnership to build AI, machine learning applications for investment management