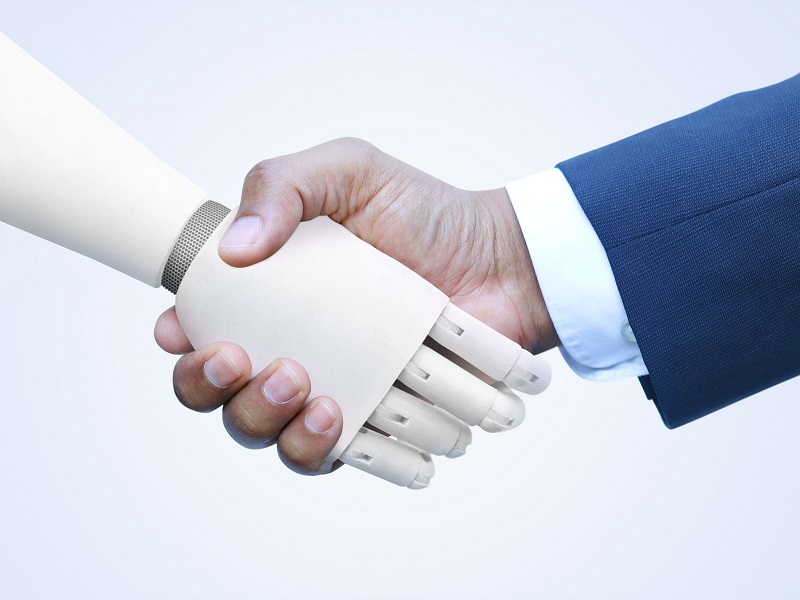
The future will increasingly favour institutional investors that can break old patterns of thought.
As we move forward and industries and new technologies converge at an increasing rate, the need for a shift in mindset is imperative. One way we believe this to be possible in investing is through a multi-disciplinary approach.
Read: Can machine learning help active managers outperform passive peers?
By combining the power of human ingenuity and continued advances in technology and data, the aim must be to construct portfolios that are more resilient and adaptable in an ever-changing world. This process requires portfolio managers to become the point-of-connection between humans and machines.
Fundamental analysis — art meets science
Most investors are familiar with fundamental analysis — a tried and true approach to identifying mis-priced securities through the study of quantitative and qualitative components. This can generate deep insights that are a function of decades of meetings with management teams and pouring over financial statements. It generally relies on assumptions formed through analysis and intuition, making it susceptible to linear thinking and both emotional and cognitive biases that can lead to inaccurate forecasts and portfolio exposures.
In short, analysts can become blind to change and can be unwilling to re-frame and update their thesis. In a purely fundamental process, this and other biases can go unchecked by the portfolio manager who often also carries their own biases on the analyst and the name being recommended. It can therefore become increasingly difficult for the individual responsible for portfolio construction to correctly narrow down on the appropriate signals.
Read: Machine learning can help with stock selection: study
Similarly, fundamental analysis can only generate strong signals for small subsets of an overall investment landscape. It certainly has a place in portfolios given its long-standing history of success when correctly applied; however, at the portfolio construction level, we believe the offsetting attributes of quantitative approaches provide a better portfolio overall.
Quantitative analysis — science in need of artists
Quantitative finance is generally viewed as a more complex field that typically makes use of advanced modelling techniques and large amounts of data to quickly generate new ideas. There are generally two distinct parts to this type of investing: research and implementation. Research generally relies on devising computational techniques to take data from a variety of sources and isolate tradeable factors. These factors are then used to implement portfolios with characteristics that are deemed attractive given the specific market environment at play.
While typically faster and unbiased at generating tradeable actions, these strategies often remain blind to regime shifts, one-off events and struggle in areas that lack accessible data. They are also susceptible to falling prey to a more-is-better approach, where too much data is mined, resulting in erroneous signals through non-efficacious models. While helpful in analysis, the data must also be properly assessed by people via robust processes to ensure the predictions have strong economic rationale.
Read: AIMCo enters partnership to build AI, machine learning applications for investment management
Technology advances have enabled many seemingly human facets of investing, such as the information gleaned from face-to-face meetings and management transcripts, to now be obtained through advances in machine learning. A recent article in the Harvard Business Review by Robert C. Pozen and Jonathan Ruane stated that while artificial intelligence and machine learning are exciting avenues for any asset manager to deploy, “[the] bottom line is that while machine learning can greatly improve the quality of data analysis, it cannot replace human judgment. To utilize these new tools effectively, asset management firms will need machines and humans to play complimentary roles.”
Figure 1: Prospering in the face of exponential change
Quantitative analysis | Fundamental analysis | |
Overview | – Seeks to understand security pricing through use of computational techniques – Aims to represent reality in terms of quantifiable values | – Seeks to understand security pricing through both qualitative and quantitative techniques – Aims to represent reality through intuition and numerical values |
Pros | – Can analyze a broad investment universe quickly | – Can generate deep forward-looking insight (especially during a regime shift) |
Cons | – Still lacks in generating insight during sudden regime shifts (although technology is advancing rapidly) | – Covers a smaller universe – Slower at uncovering actionable ideas – Susceptible to human error/bias |
By combining both quantitative and fundamental analysis, investors can reap the rewards of both processes while offsetting the downsides implicit in each. Those lacking quantitative measures are unlikely to keep pace with the same efficiency in the future, while those lacking strong fundamentals will remain hamstrung in their efforts to keep on top of increasingly fluid developments and rapidly evolving trends.
Read: Machine learning can help with stock selection: study
As my colleague, Dashmeet Singh, portfolio manager and director of quantitative research and risk at Picton Mahoney Asset Management, puts it: “Historically, quants applied a small edge over a larger breadth while fundamentals leveraged their deep edge on a smaller breadth. I believe that with the democratization of data (quants deepen their edge) and availability of technology (fundamentals increasing their breadth with more efficient tools), combining quants and fundamentals will become even more powerful than any one on its own.”